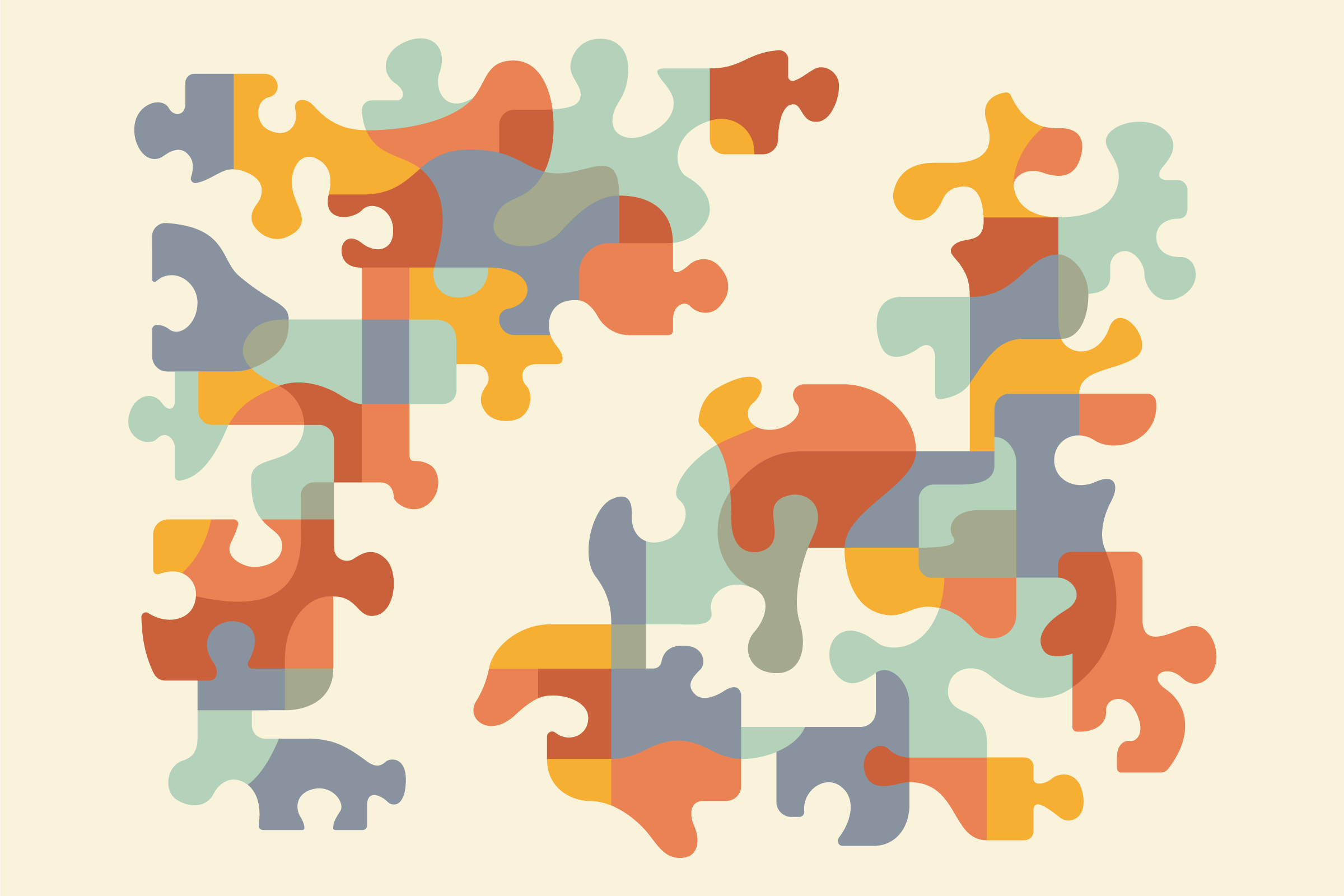
Summary: We analyzed the over 1,450 submissions to the National Telecommunications and Information Administration’s request for comment on AI accountability to identify patterns in today’s current AI policy landscape. Our primary observation is that, but for a small number of astute comments, a good deal of today’s AI policy proposals are unlikely to be effective and are rarely matched to the harms they ought to prevent. We present a simple framework that looks at the alignment between market incentives and individual benefit to identify appropriate policy interventions.
We augmented the bulk data download from regulations.gov for our analysis and have made our dataset publicly available here.
Today’s AI policy landscape has a coherence problem. There are plenty of ideas, a surfeit of headline-driven urgency, but not much of a vision for how these ideas might work together or whether they’ll work at all.
The NTIA’s RFC for AI accountability all but confirms this. The more than 1,4501 comments from companies, industry groups, nonprofits, academics, and unaffiliated individuals paint a picture of concern, thoughtfulness, but insufficient attention to the central question of what makes for a regulatory framework that actually protects individuals while allowing us to realize AI’s promise.
A simple framework for AI regulation
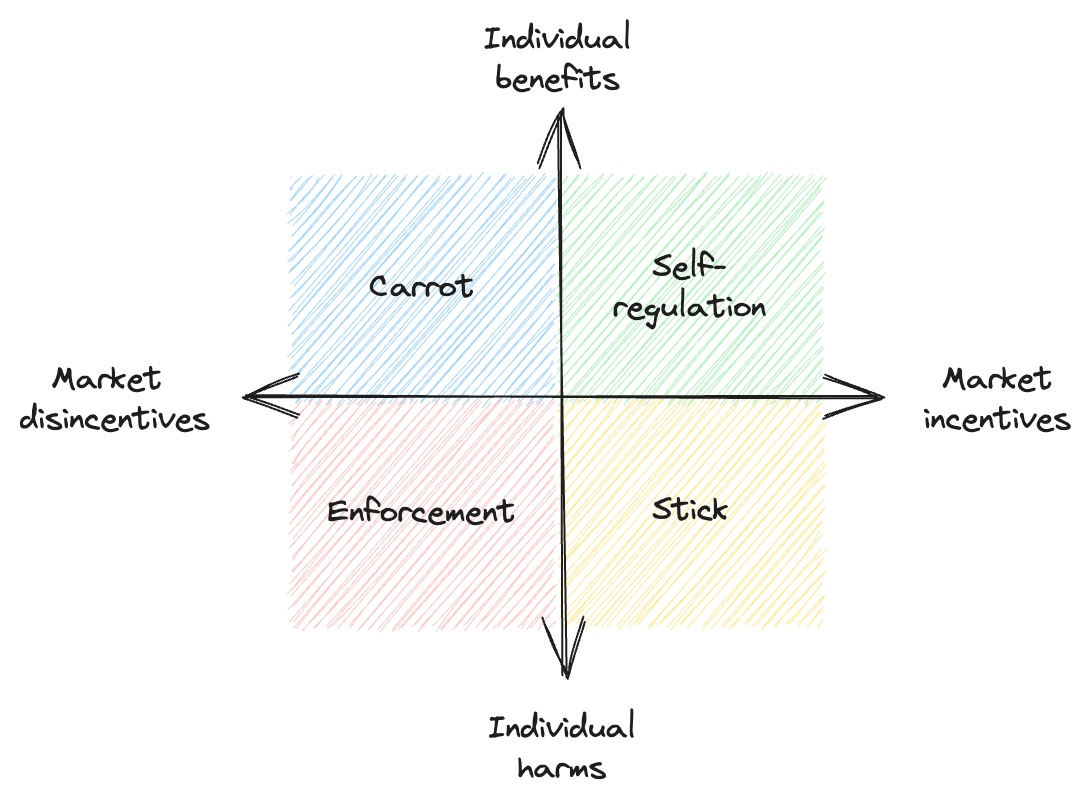
A simple but powerful way to target where regulation can be most effective is to look at where market incentives and individual benefits diverge. When they’re aligned, we can generally expect self-regulation to work, but when they part the story gets more complicated. If market participants have an incentive to do something harmful (e.g., polluting the local river) we typically need rules and enforcement. If the public would benefit from something that doesn’t make business sense (e.g., broadband in remote areas) we need encouragement and subsidies. And for things that no one wants, it usually falls to governments to prevent them.
While the framework in Figure 1 helps determine the type of regulatory solution that is needed, even a properly targeted policy must still address real harms and use the full range of tools at its disposal to be effective. We mapped the submitted comments to this framework to understand what proposals would be most effective and where there were gaps.
While some of the best ideas came from expected sources, like leading academics and companies with strong public mandates, they also came from non-technical sources – a challenge to the idea that technical AI expertise is a prerequisite for participating in AI policy debates.
Themes from professional commenters
Professional commenters, such as corporations, industry groups, non-profits, and academics had much less diversity across comments than we might have expected. Beyond a general sense of urgency we did observe a number of general themes, which we highlight below.
Government as regulator vs. enabler
A surprising number of submissions,2 particularly from corporations, assumed that the government’s primary role would be to support their best practices (audits, transparency reports, etc.). This is reasonable when behaviors fall in the “Self-regulation” quadrant of Figure 1, but certainly not in the “Stick” (or “Enforcement” quadrant) where company behavior needs to be policed. Consider sophisticated uses of private data to make targeted recommendations appear as the correct response to prompts: without privacy laws, there’s little to constrain things like these.
Mismatch between remedies and harms
It was also striking how many corporate proposals simply assumed that their proposals were beneficial without identifying the specific harms they were supposed to address. A few academic commenters highlighted the limits of this approach – both the risks of overreach and of the ineffectiveness of “AI half-measures.”3 The better proposals offered robust frameworks to address actual concerns (like preventing the proliferation of non-consensual intimate imagery), which helps to link technological advances, deployment best practices, and government enforcement to create solutions that are far more likely to succeed.4
Risk-based regulation as a near corporate consensus
Corporations were very likely to recommend “risk-based” regulation as the optimal approach for managing AI’s concerns.5 This isn’t surprising – risk regulation focuses on impact assessments and internal risk mitigation at the expense of tort liability and individual rights, which certainly favors corporate interests. As we have highlighted before, there are good reasons to reject this approach: making companies responsible for the consequences of the things they build is a much more powerful restraint than, say, an audit requirement.
Emerging confidence in NIST and similar frameworks
Where the National Institute of Standards & Technology (NIST) AI Risk Management Framework (RMF) (or similar standard) was mentioned,6 it was uniformly positive. This is a good reason to continue investing in the organization and its efforts and for developers of AI systems to take the NIST AI RMF seriously in their work.
Predictable disagreements
While no group of submitters (corporate, nonprofit, etc.) was entirely homogeneous, there were broad and somewhat expected patterns in the comments.
Corporations and industry groups generally pushed for self-regulation7 (without specific detail about the harm that would avoid) while non-profits and academics were more insistent on legal standards and enforceability.8 Where corporations were open to government regulation, they typically insisted that it should be as narrow9 as possible, whereas non-profits and academics were more disposed to broader regulatory regimes. The obvious exceptions were non-profit and research groups whose mandates are to limit government reach.
Themes from individual commenters
The very human submissions from individuals were a poignant contrast to the abstract policy proposals submitted by the professional commenters.
The RFC process lets anyone submit a comment. So while there were the occasional flip remark or throwaway comment, the bulk were expressions of genuine and often specific worries about what AI will mean in these individuals’ lives. If we want to take addressing real harms seriously, this list can act as a litmus test for proposed policy frameworks. Regulators must also make sense of future harms, identify issues not brought up by commenters, and to provide emphasis and solutions appropriate to the nature of each concern, but this is a powerful starting point for that work.
List of concerns organized by theme
There were over 1,200 individual comments, including many thoughtful policy suggestions and articulated concerns. The majority touched on the following themes:
- Economic disruption: job loss and inequality, loss of livelihood and purpose, financial hardship, copyright infringement10
- Bias and discrimination: AI bias against minorities, discrimination perpetuated by AI11
- Safety and aligned values: AI harming or manipulating humans, AI lacking human values12
- Malicious or misleading uses: spread of misinformation by AI, deepfakes, unauthorized use of likeness13
- Loss of privacy and misuse of personal or restricted data14
- Inappropriate regulation: unaddressed harms, hampering progress15
Where we go from here
This RFC is a remarkable snapshot of the AI policy landscape in June 2023. Anyone could make a case and it seems like every major player did, giving us a view into the policy intentions of the most significant and influential players in the AI ecosystem. On the other side, concerned individuals were able to express their worries about AI in their own words.
We now have a map of the AI policy landscape as expressed by its constituents – and, by extension, the expected regulatory battle lines. Now is the time to give these perspectives the scrutiny they deserve and draft rules that actually protect individuals while AI pursues its forward march.
If you would like to conduct your own analysis of the RFC, we’ve made our dataset publicly available. And if you’re interested in collaborating on the development of effective AI policy, regulation, and safety best practices, feel free to reach out and say hello.
Appendix: The view from Congress
Senator Richard J. Dubin submitted a short letter on behalf of the Senate Judiciary Committee that gives some visibility into how the branches of government are likely to interact as AI policy develops. It critiques the consequences of Section 230 of the Communications Decency Act (”…[it’s] unquestionably responsible for many of the harms associated with the Internet…”) and then goes on to say:
…[an AI] accountability regime also must include the potential for state and federal civil liability where AI causes harm… we also must review and, where necessary, update our laws to ensure the mere adoption of automated AI systems does not allow users to skirt otherwise applicable laws (e.g., where the law requires “intent”). We also should identify gaps in the law where it may be appropriate to impose strict liability for harms caused by AI, similar to the approach used in traditional products liability. And, perhaps most importantly, we must defend against efforts to exempt those who develop, deploy, and use AI systems from liability for the harms they cause.
References
-
The comments can be viewed or downloaded at regulations.gov. The official tally puts the document list at 1,476 but we noticed a few duplicates, throwaway comments, and deleted entries, bringing the number closer to 1,450. The breakdown, based on how we classified commenters using submitted information, was 52 corporations, 31 industry groups, 76 non-profits, 27 academic groups, and 1,262 individuals, as well as a congressman and the Senate Judiciary Committee. In some cases, an organization’s comment was submitted by an individual under their name rather than the organization’s – we tried to spot these and classify them correctly. ↩
-
Consider Adobe’s comment (emphasis added): “Adobe recommends a flexible approach to trustworthy AI that incentivizes AI accountability mechanisms guided by both government-developed standards and general principles of accountability, responsibility, and transparency.” Or ADP’s comment: “Self-regulation or soft law is the most appropriate way to drive AI accountability in the near term, as the field remains nascent, accountability measures continue to develop and improve, prescriptive regulation may slow innovation, and formal requirements may become quickly outdated. In addition, AI systems are subject to existing regulation, including but not limited to laws against bias and discrimination.” ↩
-
From Neil Richards, Woodrow Hartzog, and Jordan Francis of the Cordell Institute for Policy in Medicine & Law at Washington University in St. Louis: “Audits, assessments, and certifications might engender some trust in individuals who use or are subject to AI systems, and those might be helpful tools on the road to fostering trust and accountability. But there is no guarantee that trust created in this way will be reliable. Mere procedural tools of this sort will fail to create meaningful trust and accountability without a backdrop of strong, enforceable consumer and civil rights protections.” ↩
-
A good example, but not a submission to this RFC, comes from Sayash Kapoor and Arvind Narayanan. ↩
-
Interestingly, the exact wording of “risk-based” was used by 20 corporations (with even more referencing the approach but with different phrasing, like “risk management” – e.g., Microsoft). Of the 20, we are the only company that was opposed. ↩
-
NIST was mentioned with considerable frequency: 27 corporations (including the largest companies like Microsoft, Google, and IBM, as well as major AI research labs like OpenAI and Anthropic), 18 industry groups, 32 non-profits, and 10 academic groups. ↩
-
Consider Johnson & Johnson’s comment: “Industry should be encouraged to self regulate: companies should establish and implement guiding ethical and governance principles that apply throughout all their operations … We support accountability models that incentivize the detection and remediation of errors, bias, and other risks (“find-and-fix”) over approaches that penalize the discovery of such errors (“find-and-fine”), in the absence of significant wrongdoing.” ↩
-
The Cordell Institute again: “By contrast, legal standards and enforceable risk thresholds are substantive rules that offer a much better chance of successfully implementing AI systems within human-protective guardrails.” ↩
-
Consider ADP’s comment: “Our comments open with an overview and discussion of the key elements of an AI accountability system. These elements should be addressed via a self-regulatory approach such as NIST AI Risk Management Framework, which ADP is implementing, along with a consistent approach to external oversight. In the event policymakers consider more formal regulation, these elements should inform their thinking.” ↩
-
Example comments discussing this theme: 0004, 0011, 0015, 0083, 0937, and 1347 ↩
-
Example comments discussing this theme: 0058, 0342, and 0398 ↩
-
Example comments discussing this theme: 0008, 0061, and 0106 ↩
-
Example comments discussing this theme: 0018, 0098, 0179, and 0797 ↩
-
Example comments discussing this theme: 0020, 0024, 0046, 0076, and 1131 ↩